Machine Learning and Customer Segmentation / Meet the Perfect Couple
The rapid technology development is revolutionizing almost every area of business operations, including customer segmentation. Machine learning hand in hand with AI is emerging as one of the most interesting trends, transforming the way in which you can segment and, consequently, successfully reach your customers. You’re about to see how machine learning is changing the rules of the game and taking your company’s data usage to a completely new level.
What Is Customer Segmentation?
Customer segmentation is the process of dividing customers into groups according to specific characteristics, needs and behaviors. This is based on the assumption that customers in a given group will share similar reactions to marketing and sales activities. In this way, the business can adjust the company’s strategy to suit the needs of individual customer groups and successfully tap into the limited resources, rather than “burn” the budget for marketing activities that fall short of expectations.
Today, we can distinguish two customer segmentation approaches: the traditional one and that based on machine learning tools. You won’t be surprised if we say that the latter method is much more accurate. 🙂 ML can help you carry out a more in-depth analysis of your customers and manage this data with ease. You will better understand consumer expectations, define the segments of customers who are likely to churn, as well as the segments of the most loyal customers and frequent buyers.
Like a turbocharger in a car, machine learning significantly increases the efficiency of your engine, or the system for collecting and analyzing consumer data.
However, before we move on to discuss the role of machine learning in customer segmentation, let’s take a look at the traditional approach. Why do we need any technology for that anyway?
Customer Segmentation – The Traditional Approach
Customer segmentation is nothing new. Companies have been doing it for many years. Analysts rely on the good old Excel or Power BI to divide customers into all customer groups according to specific categories using sales data from Google Analytics and other sources.
The output of this exercise usually includes:
- Demographic segmentation: here we group customers/ users based on measurable parameters such as age, income, education, and occupation.
- Geographic segmentation: here customers are divided based on their location (e.g. city, region, or country).
- Psychographic segmentation: this approach divides customers into groups based on their interests and lifestyle. The data can be sourced, for example, from surveys, focus groups or in-depth interviews.
- Behavioral segmentation: finally, customers can be segmented based on their behaviors, including knowledge, attitude towards the brand, interaction with the company and products/ services purchased. The RMF analysis is a popular method that can be employed here, as it categorizes customers based on their spending history.
If you want to exploit the full potential of customer segmentation, you need to consider all four aspects. Done this way, however, the segmentation has some barriers that are difficult to overcome using the above conventional analysis methods.
Limitations of Traditional Customer Segmentation:
Too Broad Segments
Traditional customer segmentation often leads to overly broad customer groups that do not fully reflect the complexity of the customer base. For example, two customers from the same demographic group may have entirely different needs and preferences.
Not Dynamic Enough
Traditional customer segmentation is generally static and fails to note that customer behaviors and preferences tend to change over time. For example, a customer’s shopping habits may change depending on the season, time of the year and other factors that are difficult to capture at first glance.
Relying on Limited Data
Traditional segmentation methods tend to focus on superficial features and ignore the deeper insights that can be gained from analyzing transaction history and website behavior.
A Lot of Time and Effort Required
Last but not least, traditional segmentation can be time-consuming and resource-intensive, especially in the case of a large customer base. It will also be prone to bias and misjudgment.
How to deal with those limitations? Well, enter machine learning (surprise!). With this technology, your business can classify customers more effectively and accurately. How is this possible?
The Perfect Couple – Customer Segmentation and Machine Learning
You may already know what machine learning is (after all, there’s been a lot of hype around AI/ML for months), but here’s a quick reminder to be sure: 😉 ML is an AI-based technology that relies on algorithms to learn from available data to discover patterns and trends that could be used to make decisions and/or forecasts.
Here’s an example: let’s say you want to identify unapparent relationships between shoppers, such as what products people buy in a given season/ time of the year, taking into account their gender, historical purchases or margins. The algorithm is able to find groups of users who are most similar based on their characteristics and shopping history. This happens automatically. You don’t have to input values manually, vary them or create specific filters for individual groups.
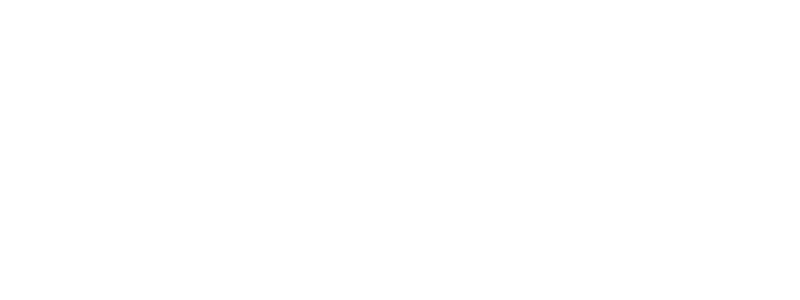
This is highly important when we are aware that different product groups have entirely different price ranges or when we have many types of customers who are valuable to us, but will have completely different spending histories.
As you can see, machine learning algorithms can analyze huge amounts of data and identify complex underlying patterns that you wouldn’t normally think of. This enables a more accurate and detailed customer segmentation.
By leveraging a variety of data sources such as demographics, spending history and products viewed, machine learning models can discover hidden customer segments and provide in-depth insights into customer preferences, needs and behaviors.
Thanks to machine learning-based customer segmentation, your business can:
- ensure more personalized customer experiences,
- stay on top of current trends among your customers,
- take better decisions based on available data.
Discover the Advantages of Customer Segmentation Based on Machine Learning
You already know how ML works and how this technology can be used for a better segmentation and strategic fit at your company. But there’s much more!
Accuracy
By leveraging the vast amount of data and complexity that AI provides, machine learning algorithms give a better insight into consumer preferences. ML algorithms search for patterns and correlations in customer data, making it possible to personalize communications, proposition, and other marketing activities.
Automatic Analysis of Vast Amounts of Data
Machine learning makes it easier to process and analyze massive data. You can have gigantic customer datasets and still be able to extract interesting insights from them using machine learning. Cloud-based tools are extremely useful here, and they can be run only for the time of calculations, analyzes and training of models.
Dynamics
Unlike traditional customer segmentation techniques, machine learning models for customer segmentation can learn from new data and adapt to changing conditions. And you don’t even have to do anything! In this way, you will stay abreast with changing trends, as you can rest assured that your understanding of customers/users is always up-to-date and correct. This is a great way to build customer loyalty and – ultimately – increase conversion.
Customer Segmentation With Machine Learning in Practice
Imagine a fashion brand selling elegant clothes for ladies. Let’s assume that this company sells both through an online store and several brick-and-mortar stores. The example is fictitious, but the business conditions are real.
Suppose our hypothetical company collects various types of customer data, including:
- spending history
- demographic profile
- behaviors related to browsing and buying products (e.g., frequently purchased products, price range, use of special deals, etc.)
Our company has decided to use machine learning to create a better customer segmentation model. Once developed, the model was trained using available data to identify distinct segments based on their customer spending patterns, needs and preferences, as well as their online behaviors. With the machine learning model, you discover two primary customer segments for the company:
- seasonal buyers
- early adopters
The first segment includes customers who regularly buy products from certain categories. For example, summer dresses in spring, elegant blouses in autumn and jackets and cardigans in winter. The clothing company can use this knowledge and adapt its marketing strategy to fit this particular segment, recommending appropriate products and offering attractive discounts. This will cause the customer’s LTV to keep growing: encouraged by tailored recommendations, she will be more likely to return to the store.
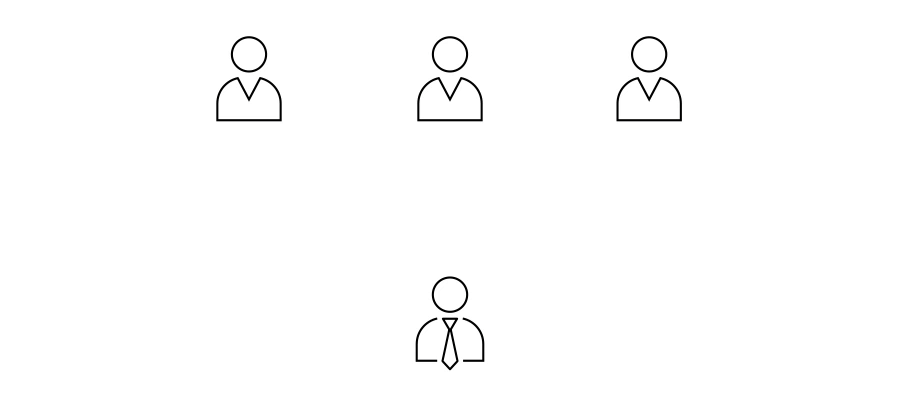
Now let’s look at the other group. Early adopters like to buy clothes that have just hit the store, and the “new arrivals” section is the first place they got to when visiting the online store or the store’s website. Again, the company can use this knowledge to devise special marketing campaigns, showcase the latest clothing collections, send personalized emails with pre-sale offers, and display relevant product recommendations on the website.
And that’s what it’s all about – you can use machine learning to reach customers more effectively, thereby increasing conversion and improving customer experience. Remember that a marketing message will eventually become a dud when sent out to all customers indiscriminately.
How to Use Machine Learning for Customer Segmentation
There are a few important things to keep in mind if you wish to segment your customers effectively with machine learning. Use the following tips to run the whole process smoothly and reduce the risk or failure or unnecessary delays.
1 / Collect and Analyze the Right Data
Modern segmentation requires an understanding of the data analysis and machine learning process. Firstly, define your business goals and create the right infrastructure to collect the data you need. To build algorithms that will identify patterns in shoppers’ behaviors and actions, you require well-prepared data, ideally collected in a data lake or data warehouse. This infrastructure allows data engineers and data scientists to analyze data more easily and quickly.
2 / Make Sure You Have Modern IT System Architecture
As you already know, to be able to perform effective data analyzes for customer segmentation, you need a single source of data. But that’s not all. You also require a system architecture where data will flow quickly and smoothly without draining internal system resources.
E-commerce platforms, ERP systems, warehouse management systems, product management systems, data from the website … information from all these systems, whether modern or legacy ones (and we have a way to deal with the latter too 😉) should be updated according to the agreed schedule and business needs. After all, some data, such as orders and stock levels will need to be updated on an ongoing basis, while other data, e.g., loyalty card data, can be refreshed, say, every hour.
3 / Use the Cloud
It will need a cloud to carry out complex ML processes and process large amounts of data. The cloud uses scalable and versatile platforms to store and process the massive amounts of data needed to train machine learning models. With embedded machine learning algorithms and analytics, these tools make it easier to train models, get new customer insights, and use them for sales/marketing. What’s more, cloud-based tools can be accessed from anywhere in the world and often have user-friendly interfaces and flexible payment methods, which lowers the entry barrier for businesses. These tools can also be run when needed, which also has a positive impact on the budget.
Challenges of Customer Segmentation Based on Machine Learning
Understandably, there is always a flip side to any coin. Customer segmentation based on machine learning comes with several challenges that you need to be aware of:
Data Security
As the focus on customer data protection becomes stronger, your business needs to ensure compliance with data privacy regulations such as the GDPR. The collection, storage and processing of customer data for machine learning models require the explicit consent of the data subjects and must have clearly defined purposes.
Experience and Technical Knowledge
Implementing machine learning models requires a certain level of skill and knowledge of data science and artificial intelligence. More often than not, these resources are not within easy reach for many organizations. Machine learning specialists are in short supply and costly to hire. In many cases, you’ll be better off working with external experts who specialize in implementing machine learning solutions for business.

Lack of High-Quality Data
Machine learning models for customer segmentation require large amounts of high-quality data for initial training. Your company may struggle to collect enough data or to ensure that the data it has is of high quality and well-structured. That is why it’s so important to prepare the right infrastructure for data collection, e.g., a data lake or data warehouse. Before you start working on your ML model, you need to make sure you have all the necessary data sources at hand. Otherwise, the whole process will last longer.
Integrating the ML Model With Other Tools
Deploying machine learning models for customer segmentation requires technical knowledge and integration with existing systems and tools. As well as being complex and time-consuming, the process will require overcoming numerous organizational barriers.
But Don’t Hem and Haw: Start Segmenting With Machine Learning Now
The increasing complexity of customer needs and the business environment requires a more advanced approach to customer segmentation. Machine learning provides the solutions you need to build a competitive edge. With this technology – specifically its ability to process massive amounts of data, its accuracy and flexibility – you can group your customers much faster and better.
Now is the best time to embrace this technology. There’s no point in waiting, as in today’s competitive business environment, keeping up with the competition is a must rather than a luxury.