How to Use AI in Business / A Practical Guide
Over the past few months, the number of AI-based tools and systems has exploded. More and more people are talking about using AI in business. However, the common narrative suggests that artificial intelligence technology is some kind of magic that solves all problems on its own, or a way to generate cool pictures. Managers and business owners have a right to feel a bit confused.
If you are feeling the same way, we are here to help you! We will explain how to practically implement a project using artificial intelligence and data science tools in your company to improve business operations and boost revenue.
Artificial Intelligence and Data Science – How are They Linked?
Data science and artificial intelligence are closely related and complement each other.
- Data science involves analyzing large datasets to extract valuable and useful information that can be translated into actionable insights and business growth.
- Artificial intelligence, on the other hand, focuses on the creation of systems that have the ability to think and learn in a manner similar to that of the human brain.
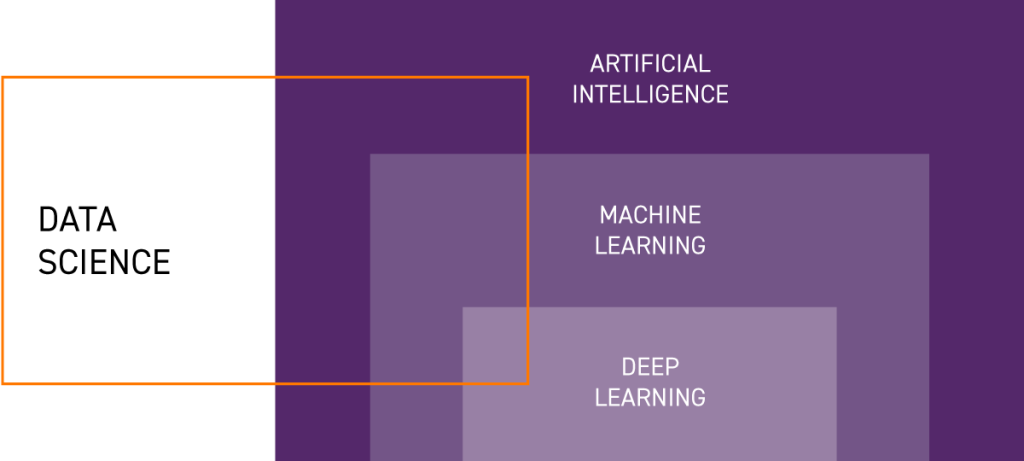
Data science uses advanced mathematics and AI tools like machine learning and natural language processing to analyze large data sets. Artificial intelligence enables data science professionals to more accurately analyze data and identify patterns that translate into real business value, such as:
- Better understanding customer behavior and recommending more tailored products
- Sales forecasting with predictive analytics
- Better understanding of market trends
- Spotting anomalies and potential problems in data
- Optimizing business processes
- Predicting customer churn
- Automating repetitive tasks
Why are Business AI Projects Different?
You may already be on your way to digital transformation and have implemented various IT solutions. Maybe it’s e-commerce platforms, product management systems, building cloud infrastructure or implementing process automation.
Now, you want to take the next step and take advantage of the benefits of artificial intelligence and data science to drive the growth of your business. You’re looking for experts who can extract interesting information from your data and translate it into real business benefits like increased revenue.
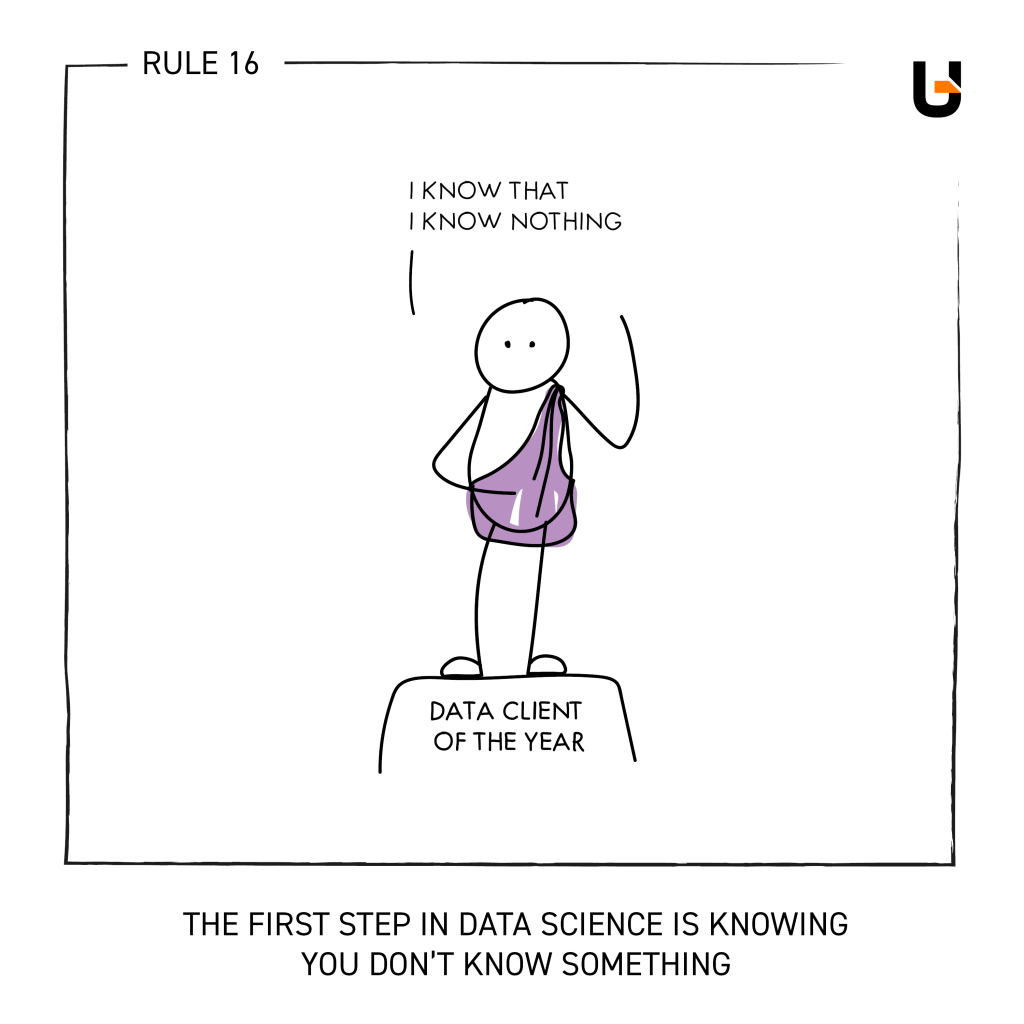
And this is where we encounter the most common mistake in approaching data science projects, which are treated like any other IT system implementation in the company.
- Data science and AI projects are similar to a science project – hypotheses are made, then experiments are conducted (initially on a smaller sample) to verify those hypotheses. You can’t be 100% sure that a hypothesis, such as a 5-10% increase in sales, will be confirmed. Maybe it will be 3%, maybe 15%, or maybe 0. Of course, there are ways to reduce the risk of failure, such as collecting data in the right way, as we will tell you in a moment.
- The work is done iteratively – if the model does not meet the objectives, it is refined and other variants are tested. Once again, the “science” element in “data science” is not a coincidence.
- The financial benefits of such a project are deferred. The full verification of the model takes place after implementation. In order to know if a data science model is going to increase sales, those sales simply have to happen.
How to Prepare for an AI & Data Science Project
To start a data science project in your organization, you need (surprise!) data. It sounds obvious, but it is what it is, and what’s more, it can’t be just any data.

Data Strategy
Before you move on to implementing advanced machine learning models, you need to become a data-centric organization and develop a data strategy for your company. Answer the important questions of “what data?” and “for what purpose?” you want to collect it.
Data Collection
Once you have a strategy, start collecting data. One solution is a data lake, which is a place to store a large amount of diverse data that can be processed quickly and flexibly. Another solution may be a data warehouse, which is a central location for storing business data that has been pre-processed and structured to make it easier for the organization to use.
Simple Analysis
You can then begin to perform basic historical data analytics using tools such as Power BI.
However, all of these activities take time, and if you are thinking about using your data and AI systems in the long term to create a competitive advantage and increase revenue, you should immediately prepare for moving on to the fourth stage: the practical implementation of artificial intelligence in business. As you will soon see, this approach pays off.
What Do AI & Data Science Projects in the Business World Look Like?
If you already have a developed strategy, as well as properly collected data that you are already analyzing, you can move on to real AI & data science projects. To help you better understand what’s going on at each stage, we’ll walk you through the process.
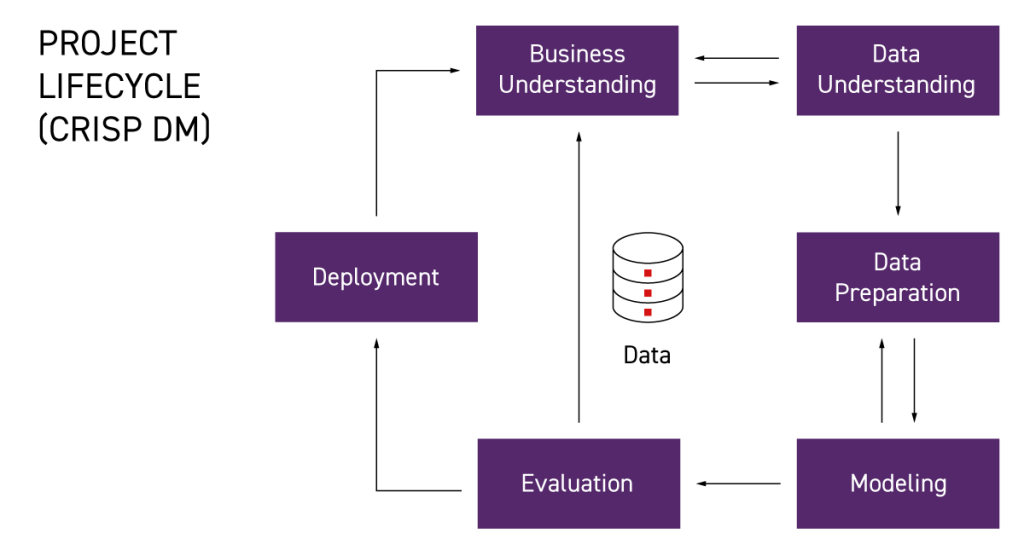
1 / Business Analysis
In the first step, business leaders and data specialists engage in a dialog to understand the business problem and find a solution using data science and AI tools. For example, your need may be to increase sales in an online store, and the AI technology that will allow you to achieve this will be recommendation systems or dynamic pricing.
In this step, it is also necessary to check if there are any legal or technical restrictions that could jeopardize the project. For example, you may need real-time data for the model to work properly, but for various reasons you may not be able to obtain it. You will find these types of difficulties at the beginning of the project.
2 / Understand the Data
In the second step, the data scientist needs to look at the data that the company has. For example, if the goal is to increase sales through the recommendation systems mentioned above, it will be necessary to look at the sales history.
Data scientists examine what data can go into the model, its quality, completeness, and consistency. For example, when analyzing sales history, it may turn out that the pandemic period should be omitted because customers’ purchasing behavior was different from the norm and using this data today could distort the model.
3 / Data Preparation
The third step of the data science project is to prepare the data for the algorithm. At this stage, the data is reviewed, cleaned of gaps and errors, and transformed into the correct form. For example, if a data scientist wants to build a sales forecasting model, he or she can do so on a daily, weekly, or monthly basis, etc. That’s how your data should be prepared for the algorithm to get the results you expect.
4 / Data Modelling
At this stage, the Data Scientist develops the right model to become the foundation of the artificial intelligence and data science solutions implemented in business, such as a recommendation system or a sales forecasting system. This is a highly technical stage, largely dedicated to the use of data mining techniques and creating machine learning algorithms.
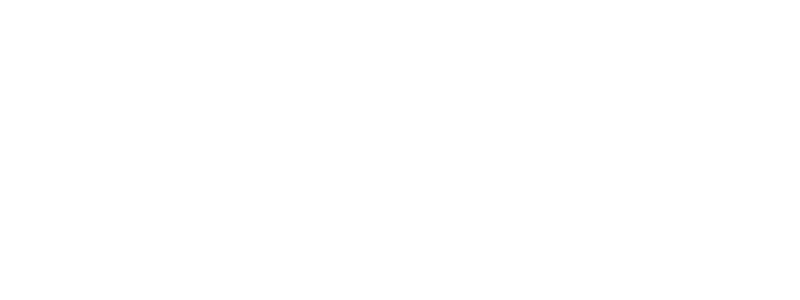
5 / Model Evaluation
At this stage, the model is tested against the assumed business objectives, for example a 5-10% increase in sales. If the recommendation engine was the chosen solution to achieve this goal, it is now running on the site for a few percent of customers. In this way, the performance of the model can be verified on a smaller sample.
If the model fails to perform as expected and needs to be improved, the process starts again. The business assumptions and data need to be re-examined to fine-tune the model. Verification may also involve business and internal experts who evaluate the model’s performance. As we wrote earlier, data science is a scientific process – testing hypotheses and finding the best solution.
Step five is also when organized, thoughtful data collection in data lakes or warehouses bears fruit. When data specialists have access to a centralized data source, data processing and hypothesis testing are much faster and the risk of failure is lower. The model can then be built and evaluated in a matter of weeks. As a result, subsequent versions of the model can be tested more quickly.
6/ Full Implementation
In the last step, when the company is satisfied with the results and the evaluation of the effectiveness of the model on a smaller sample, an IT system is created that becomes part of the company. For example, the recommendation engine is implemented for all customers and the magic begins to happen. 😉 Sometime after the implementation, you can fully evaluate the effectiveness of the model and the system as a whole. As we mentioned, if the goal was to increase sales and boost revenue, then those sales have to happen to count if they actually increased by the assumed value.
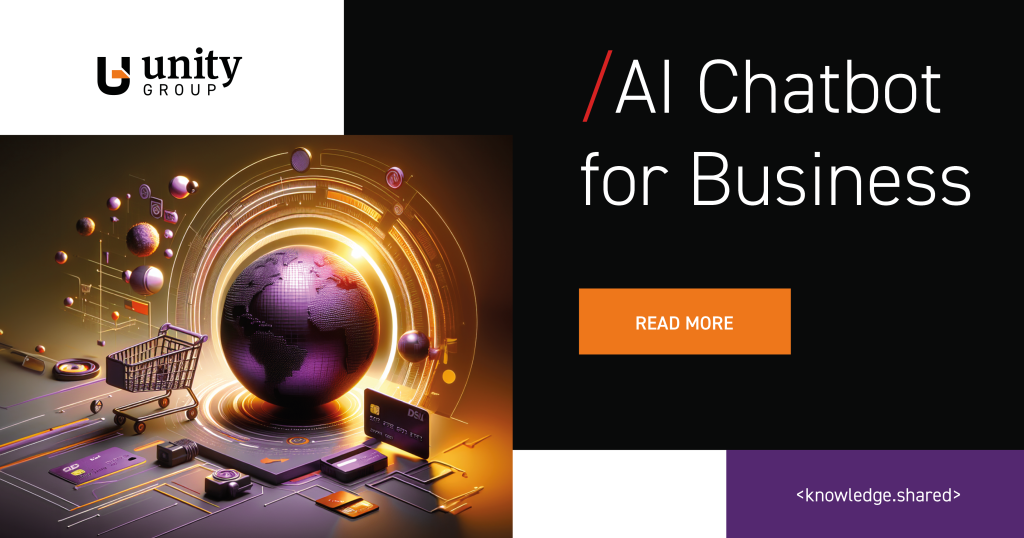
So, You Have Implemented Artificial Intelligence in Your Business. What’s Next?
Besides the fact that you have a lot to be happy about, you need to know what to do next 😉 From time to time, your algorithm will require periodic reviews for KPIs and business metrics. Some changes to the model itself may be necessary. As you already know, solutions based on artificial intelligence and data science require good quality data.
But data often changes. Maybe your company has expanded abroad, where the market looks completely different? The model needs to be updated with new information.
AI & Data Science Magic is Really Science
Today, the path of digital transformation has to lead to the use of data in a smart and strategic way. In the near future, the competitive advantage will be built by those who can use data to grow their business. The way to achieve these goals is through projects that use data science and artificial intelligence solutions. It is worth remembering that these are not magic tools that we implement with two clicks. It is the result of a scientific, iterative process – data analysis, testing, verification – that leads to business growth.
Do you want to unleash the power of your data?